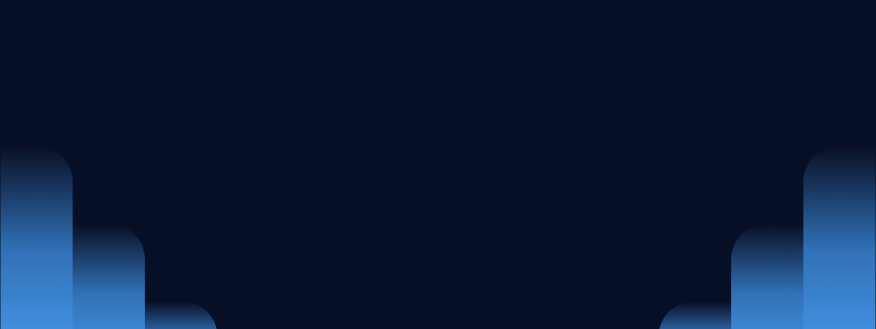
Interview Mind Foundry
Mind Foundry was founded about 8 years ago by two professors of AI from Oxford University in the UK. Oxford University is probably the Premier University in the UK and has globally the highest number of successful spinoffs in terms of companies in technology and other areas ahead of Stanford and others in the US.
It's been a very successful incubator for new startups.
Now when Professor Mike Osborne and Professor Steven set the company up, they had been working in the sphere for 20+ years and they still are professors at the university within AI. They had 3 fundamental principles of AI, and these principles have never changed:
The first principle is transparency. Everyone needs to understand how it works and why. That's the only way you engender trust in using it going forwards.
The second is human AI collaboration. Humans have to be able to use it effectively in their work and derive value from it through their work. Otherwise, again, it will lose trust and won't be used.
The third one is a little bit more esoteric, and it's the thing called continuous meta-learning.
Now fundamentally for Mind Foundry, we're focused on a thing called high-stakes applications. These are areas of business globally that affect either individuals to a high degree or at the scale of populations. Something you implement or do affects a whole population.
So that's driven our investment in three key verticals, which are insurance, infrastructure, and defense. These are areas which we think have high stakes. Very importantly, it means that transparency and human AI collaboration are critical to that, especially in high-stakes applications.
For us, for example, we don't typically get involved in customer service applications and marketing applications that have less impact on the individual in high-stakes situations. The vision statement for Mind Foundry, and so that is our goal. That's been our mission.
With this remarkable growth in AI funding, how does mind foundry perceive the market opportunity for insurers currently in adopting AI technologies? And what is the unique value?
For us, we've looked at what we think the high stakes market is. It's very hard to put an absolute number on it, but if we anticipate around 20% of the whole AI market, high stakes would include insurance and defense. 20% is still a very, very large number. It's in the right, but sort of in the $90 billion range or something. And I said there's still a giant number that we can go after in that market.
We did a lot of research in the market over the last six months, we've asked all the largest insurers in the world where is your focus, where's your focus on AI specifically? What problems do you have with it and where do you see it going next?
Now, fundamentally, we've learned that the most mature, consistent place for AI is in pricing because insurance is a very price driven product. Whichever way, whichever part of the world you are. That’s where most of the investment has gone into AI, very closely followed by claims and counter fraud, to drive differential value. And so those are the classic three areas where I see a huge amount of investment and money and time to drive differential value in those marketplaces.
Now what's also interesting to me is a longer tail that's the value that will come later. And you will see investments in things like finance, customer service or supply chain a lot more, so focused on organizational control and management.
Further insurer research from Mind Foundry: Today the number one ranked concern amongst all the insurers is the governance of AI models. For instance, just in pricing today there are more than 300 pricing models. Can you control, explain and make those transparent or retrain the models effectively? That’s very difficult to do and that’s why we're coming in and building out solutions helping insurers in the governance of those AI models.
So the real problem insurers have when they’re trying when entering in AI is how do you scale it efficiently without having lots of manual costs.
The other number that came out of our research was that 50% of a data science team was spent governing those models. So rather than building new models, they’re explaining how the price came about, retraining the models, making sure they work effectively, checking to see if private data has been used and GDPR is being controlled effectively…
This takes up an enormous amount of time for these teams, and that is the real blocker towards some of these organizations to really scale further to the next level where AI becomes a continuous operation throughout their entity, with individual AI learning agents everywhere, essentially running the operation.
You can't get to that until you have the platform that effectively you believe in and the regulator understands and trusts and the customer does until the insurer gets that level, the manual approach is acting as a blocker to scale AI.
We’re seeing important concerns on technology adoption, the speed of technology exceeds the speed to adopt it, how to solve this problem?
Addressing the focus on Gen AI, the biggest driver, of course, is that it’s so accessible, so every person in an organization can immediately see how it can potentially add value. They might not understand how it works or where the concerns are, particularly around it, but they immediately see how it can make things easier and better.
The first step for a company concerned with experimentation is to immediately create a walled garden. The staff and employees and contractors can experiment safely without exposing their internal data.
The second point is that once they've done that then people are experimenting with it in areas that have been perceived as difficult to automate, typically in areas like claims, where you've got lots of bespoke processes within the organization that grow up over time and perceived as best practice internally but they're really hard to scale and cost money. Here generative AI can certainly solve some of those issues but problematically these are opaque models where it is difficult to explain how they work or act.
That’s why we believe that we can help organizations to solve issues or processes that have a regulatory impact because you cannot explain or act on opaque models. It is impossible to make a regulated decision because the regulator would say, well, can you explain how that came about and the reply is well, no, not without going to one of the vendors and asking how their model works.
And this is the real question that a lot of senior leaders in the insurance industry are grappling with. They've said to me, help us realize the value of this very low-cost technology but in a way that doesn't break our business or expose it to regulatory or reputational harm. And that's where a lot of the investment now is going into Generative AI.
We're working intensely on AI governance generally: we're doing work for insurers at the moment in our governance platform. For Generative AI specifically the questions include Where do those guardrails come in? Can these guard rails be controlled? Or is it just the fact that you can only use it for certain levels of interaction, and it combines with a trained model in order to make a regulated decision?
So, the reality is that insurers are generally aware of the first problem which is controlling their employees in the use of generative AI. The second concern is how do you then roll it out. There's a dream of the industry, which I think is probably far off, that eventually you could remove 90% of your claims handling cost by using generative models to entirely collaborate and talk to the customer. That's a very large prize, and that prize is driving a lot of the industry to look at these approaches in more detail.
But there is a fundamental issue with generative AI, as we know, that you cannot explain and control those models enough to avoid regulatory and reputational damage. So I think that whether the industry solves this key issue or not, the acceptable degree of risk to accept is the big question.
What are the best practices that you have identified for the AI implementation?
For Mind Foundry, it's all about what we call the engagement proposal. So before you do any actual work, we spend a lot of time upfront getting into the guts of what the problem is. Speaking a lot to the business leaders in those organizations, the technology is important, obviously, but more important is what's the problem you're trying to solve and what's the detail behind it? What have you tried before? What works and doesn't work? What, in the first wave, would you be likely to adopt and work with? What wouldn't you trust versus trust?
It's an incredibly detailed process, but essentially, we try and do that for a very long period before really getting to look at the data first. Have they got the data in the right state in the right place? And that's a big issue in insurance. You know, it's a very prosaic thing and lots of insurers haven't yet got all of their data in one place that can be used effectively for building these models. So that's step 2.
Step three is beginning to work iteratively and agilely with them to build out a model that actually solves a real problem, can be used by them, and is trusted by them, the regulators, and the consumers. And then, see real value.
So I think we're a bit unique in that we almost temper the enthusiasm for the technology by saying right, let's get to the heart of what this is first and then be really clear on the end goals and the value it's going to deliver and then measure the success of it against those things. Who's going to own it? Who's going to be the person? And it must be the skilled people at the insurer.
You can’t really embed any of this technology into their operation if it's very third party and kept at a distance and not really understood and not transparent and doesn’t have human AI collaboration at the heart. If these conditions are not met eventually, it will break very quickly. They'll lose trust in it and they won't use it. So, all those things must be true in order for it to be an integral part of what they do.
In this type of AI technologies that are going to change so quickly, how can companies tackle it?
If you look at Wejo going bust last year, they were burning $250 million a year, they were one of the first interconnected car data aggregation and their revenues hit $8,000,000 by the time they went bust. You've got to absolutely align market needs with the solution. I wrote a paper in 2012 saying connected car data was coming quickly and that would be the next source of crucial insight into how consumers behave. But it has taken much longer than expected to get here.
Last year, several large organizations paused their investments in connected car and market rollout to try and align the timescales. So yeah, it's absolutely a commercial reality. How much money you put into technology. The early players do tend to suffer. They go all out on the technology, and then the market says I'm not ready, especially in insurance, which is a relatively slow adopter.
A merit-based reward system has garnered attention for its impact on customer behavior. We’ve seen some models from tech leaders like Tesla failing in this approach or some models like continuous underwriting in Life Insurance with still pessimistic opinions. How can you maintain this risk evaluation approach with customer centric activities, operations in claims and pricing strategies?
I think the first thing to say is it's hard to be generalist about this as every country has a different cultural and technological landscape for IoT.
The US is going very well; 50% of all new policies in the US now are, certainly in the top 20 insurers, telematics policies. The current challenge is the cancellation rate that is very high, around 25%. They're being cycled back onto a non-telematics policy. What that tells you is that customers are not homogeneous. They're heterogeneous in terms of how they think about being monitored and the value they get from the technology.
We must put them into different buckets. Some hate it, don't want to be monitored, just want insurance to take money from them once a year and then on average pay a claim every 15 years. Others are willing to take a cheaper price to be monitored but don't want constant monitoring. Then there are those who want intensive monitoring paired to an app, to tell them about various things, help with parking, integrate different data sources, etc.
There's a continuum of people, and that varies by country, although not as much as you think. It used to vary by age, but now it's fairly flat across different age groups. When you're thinking about the technology, you must consider the cost of deploying it, monitoring it, measuring it, and managing it. How do you deploy it in a way that excites those customers who are interested, drives up retention, drives down claims, increases purchase of add-ons, and other ancillary products without harming your business?
It will be slow, it won't be like a Big Bang. It will be incremental as costs come down, data improves, and trust in the technology improves. It's not for everyone; it's for certain groups of people that respond positively to that type of interaction.
Besides the successful Driving Behavior model, can you share other notable use cases where Mind Foundry’s AI technologies have demonstrated significant accuracy and positive outcomes?
For us, we're working on several things with big insurers, helping them build out very AI-driven models around understanding customer behavior and providing the right messaging at the right time.
In the short term, building AI models for fraud detection is interesting. We've deployed a solution in the UK market, seeing a 4% reduction in claim indemnity spend, that’s a massive number for a fraud solution. And that’s because we spend a lot of time with clients understanding exactly how they would use it, encoding the experience of their investigators into the thinking behind the algorithms and deploying in a way where before you even look at a single fraud referral you can click on it and see exactly what made the referral. That’s the most powerful factor that drove that decision because investigators can agree or disagree with that referral and decide how to take it forward. So we’re not working to automate the whole process but to help human investigators to make much better decisions in every insurance organization even in the large ones. If you can make those people more effective, you will save a lot more money and you’ll also get a better reputation on fraud detection.
We’ve deployed the continuous meta learning model. That is essentially a patented technology that takes an existing model and an existing set of data and then tries to think of a new way of using it automatically and presents that back to the human operators to say would this be useful.
We've also worked heavily on retention models, seeing between 2 and 4% retention improvement. It doesn’t sound large but actually it’s quite big because it’s pure profit to the insurer. The key is helping humans make better decisions in every insurance organization.
Additionally, in the long-term we've applied quantum computing to insurance, working closely with qubit manufacturers we’ve achieved a 100x extra speed of qubit generation using our machine learning and AI capabilities and we’ve taken that learning to insurers to say what are the best use cases for Quantum and when is it likely to occur? And we’ve found that mobility is a big thing because quantum computers are very good at looking at many billions or trillions of outcomes that could all occur and then picking out of all of them which is the best.
Taking the telematics problem and saying ok can we detect cognitive decline in aging people by looking at telematics data? So, we can get it quicker and help them to be on the road longer by making them aware of the situation so they can do something about it.
Quantum is very good at looking at many outcomes and picking the best one, making it suitable for mobility solutions and fraud detection. Addressing the aging population problem, detecting cognitive decline through telematics data to help individuals stay on the road longer has been a very successful model being deployed in Japan.
Tech Giant companies are going to influence the overall data landscape in insurance and is it necessary for differentiating to, to be in partnership with them or to have any kind of relationship or strategic collaboration with them?
The data landscape I think obviously we know what's happening there and I think there's going to be an underestimate of how long it takes insurers to change. There's still a long tail of insurers who are desperately trying to reorganize their data into very clear cloud entities with entity resolution across their different people or companies in there and then deploying Kubernetes clusters so they can really deploy AI effectively and gain insight into that.
Others are still trying to get there because they want to reduce their costs of data management. They want to increase their quality and increase their deploy ability across all the different use cases. Generative AI will be a key driver in investment in that wall garden environment, but you can only really make that happen if you have organized your data internally.
To effectively do that, I think in terms of differentiation is partnering with insurers and tech giants. Tech giants are not very vertically aware, so don't understand in detail what the insurance problems are, how the insurers work. It's hard to do this stuff and whilst they are very good at being tech vendors, they need partners who can help them really penetrate the insurers and understand their problems and make technology infrastructure into something highly specific that solves the problem. The three most important challenges of AI adoption, now about ethical, security and probably about their language skills, how does your company navigate and adapt to these challenges?
The compliance and innovation will continue with AI investment, for Mind Foundry in the engagement proposal process, we do an ethical scoring and analysis of any piece of work we do. We won't do a piece of work if we think it's not ethical. For us, it's fundamentally core to who we are and that has to go through a committee and non-exec directors. And in our non-exec committees, we've got people who are not only investors but also in the university sector as well.
Everything we do has to be for the right reasons. But more prosaically, in the platform approach for insurance, we allow insurers to build thresholds into the model monitoring that says, if we suddenly apply too much price increase to Group A or Group B or Group C, create a threshold and our system will flag it as a biased pricing detection. If we're using too much of a particular factor that could be problematic signal and our system will also retrain the model away from those problematic areas.
So for us, that governance control management piece is built into the platform and really critical to the way insurance does business.
And ultimately, what we're trying to do is allow people to make the right decisions for their customers and from a reputational perspective, but also to avoid some compliance problems. Every market has a different regulator or different series of regulators, and the platform allows you to flexibly deploy against those regulatory models.
Subscribe to Our Newsletter
Get the latest insights about Global solutions for leading insurers on your email