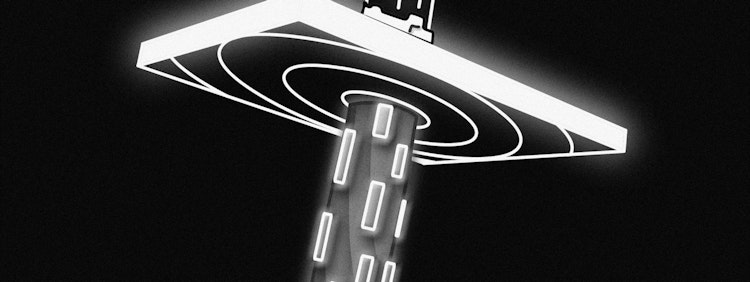
Gen AI Recap: Where Are We After the Peak of Gen AI Expectations?
Generative AI (Gen AI) is a subset of AI, but it is certainly not the same thing. Since the emergence of large language models (LLMs), the market has gone into a frenzy over a technology that has existed for decades. Take AI, add an intuitive interface, make it more accessible to consumers, and suddenly, it becomes the most disruptive technology in the world. To me, it is disruptive mainly because we’ve collectively bought into this idea.
At first, the insurance industry didn’t just buy into it. We saw Gen AI as the potential solution to everything. Gen AI for climate, cyber, and other emerging risks. Gen AI for risk assessment, pricing, and claims processing. While there was some truth to these expectations, the reality was far less precise and often superficial. How could tokenization and next-word prediction truly support the insurance industry at the level of depth and complexity it requires?
A useful way to understand the hype is this: Gen AI made AI accessible to the masses. It became "AI for dummies," allowing a broader audience to recognize its potential. For the insurance sector, Gen AI has had an indirect impact, primarily in areas like document processing, chatbots, and pattern recognition. Sound familiar?
The Shift from Hype to Practicality
Six months have passed since my last Gen AI update, and in that time, we’ve seen more clarity regarding its definition, applications, and results. I’d categorize the first phase of Gen AI (2022–2024) as a "divergence period," where organizations experimented with its application across multiple business functions. In 2025, we will likely enter a "convergence period," where companies narrow their focus to more targeted and practical applications. These applications generally fall into three key categories:
· Chatbots – AI-driven communication tools capable of resolving requests and problems by leveraging past data and pattern recognition. These solutions primarily enhance efficiency and productivity.
· Document Processing – The digitalization and standardization of data. Gen AI excels at identifying relevant information and organizing it effectively, making it particularly useful for underwriting and compliance. By comparing ideal models to actual data, it reinforces processes through rapid information retrieval.
· Pattern Recognition – LLMs’ predictive capabilities allow them to detect undesirable patterns. Fraud detection is a clear beneficiary, though traditional machine learning remains the backbone of Gen AI’s success in this area.
To put it simply: if you can imagine an application for ChatGPT in your business, that’s about the extent of what Gen AI can currently achieve. The technology in this "convergence period" is focused on structure, standardization, and automation, freeing humans from low-reasoning tasks so they can focus on higher-value decision-making.
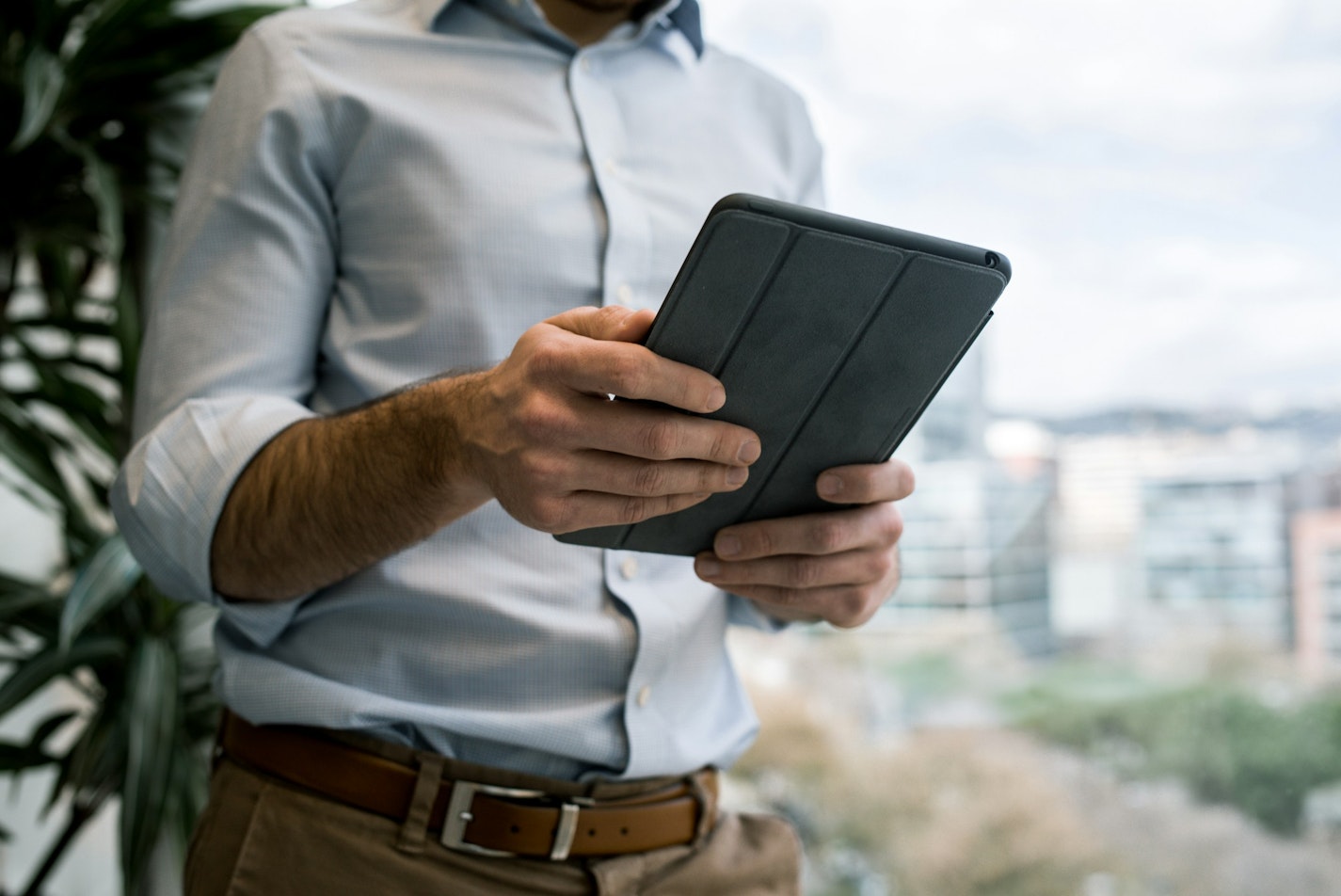
Who would have thought that past challenges in insurance, such as digitalization and connectivity, would find solutions in a technology that feels a decade ahead of its time? Ultimately, Gen AI’s biggest impact is accelerating cloud adoption through digitalization and data standardization. It enables underwriters to collect and analyze data at unprecedented speeds, automates low-complexity underwriting decisions, and translates complex data insights into business-friendly language. It enhances fraud detection through pattern recognition. In short, Gen AI is about enabling, accelerating, and improving efficiency across insurance processes.
The Next Divergence Period: Agentic AI and AGI
The next big hype cycle is here: Agentic AI and Artificial General Intelligence (AGI).
Agentic AI refers to autonomous AI capable of executing tasks independently.
AGI (Artificial General Intelligence) represents AI with cross-domain knowledge and advanced reasoning capabilities.
The Promise vs. The Reality
The promise? AI collaborating with other AI to complete human tasks at record speed and machines with PhD-level reasoning.
The likely reality?
I don’t know for certain, but it will probably land somewhere between market hype and my own skepticism. A good way to approach this is to imagine realistic use cases.
For Agentic AI, machines initiating actions autonomously will require expert oversight, less in executing tasks and more in setup and maintenance. Rather than simply instructing AI to "do something," organizations must educate AI models and grant them structured data access so they can learn what, when, and how to act. Essentially, it’s machine learning plus artificial intelligence, with greater autonomy and a user-friendly interface.
For AGI, I believe we are still far from achieving it, certainly much farther than the market suggests. True AGI requires reasoning built on data, ideas, and connections that aren’t explicitly documented anywhere. I’m particularly curious to see how leading AI companies like OpenAI and DeepSeek will navigate this challenge.
A Word of Advice
My take? Insurers should focus on adopting and refining proven Gen AI applications rather than striving to be pioneers in AI innovation. There’s no need to rush into deploying the most advanced models before their real value is clear. But that’s just my perspective.
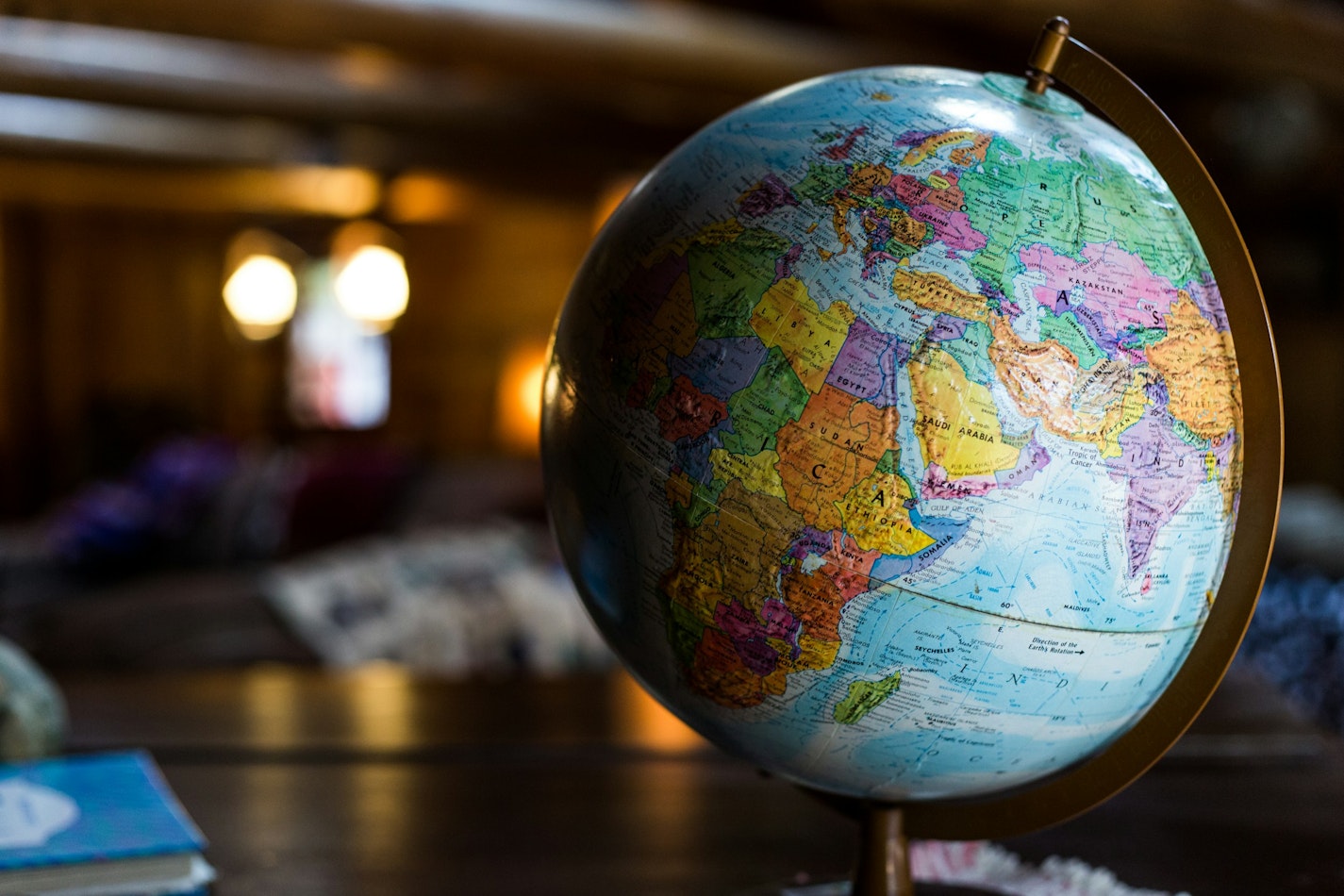
I hope my viewpoint leans toward the realistic (or even the pessimistic) side of the Gen AI debate. The market needs more critical thinking, and I encourage others to question industry narratives rather than blindly following the hype.
Yes, Gen AI is powerful, but it won’t solve climate disasters, fix the rising cost of living, or reduce wealth concentration. It won’t make insurance much more accessible, appealing, or trustworthy in the eyes of customers. But what it can do is improve efficiency, accelerate digital transformation, and enhance existing insurance processes. And for now, that’s enough.
Header photo by Google DeepMind on Unsplash
Subscribe to Our Newsletter
Get the latest insights about Global solutions for leading insurers on your email