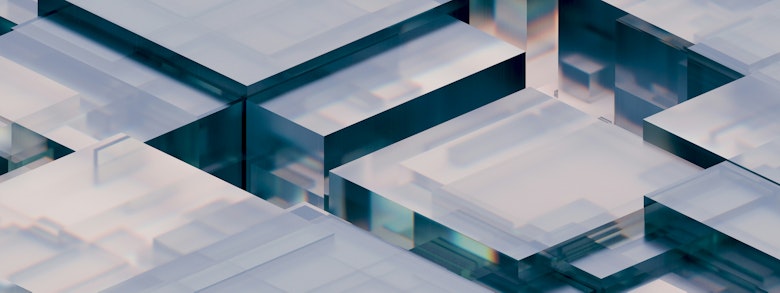
Reimagining Insurance Premium Optimization with AI and Pricing Tech
As a strategic consultant, one of the most common questions I encounter is: "How do you set the price for an insurance product?" Pricing is both a science and an art. While it traditionally relies on the classic model of supply and demand, modern pricing strategies also consider customer experience and psychological factors. Insurance pricing is no longer just about numbers; it’s also about understanding customer behavior and market dynamics.
Historically, pricing was about building models aimed at achieving market equilibrium, where supply met demand. However, with the rapid advancements in AI and pricing technology, the focus is shifting. Today, industries are moving towards an optimization strategy, one that goes beyond market equilibrium and focuses on creating a balance between profitability and customer satisfaction.
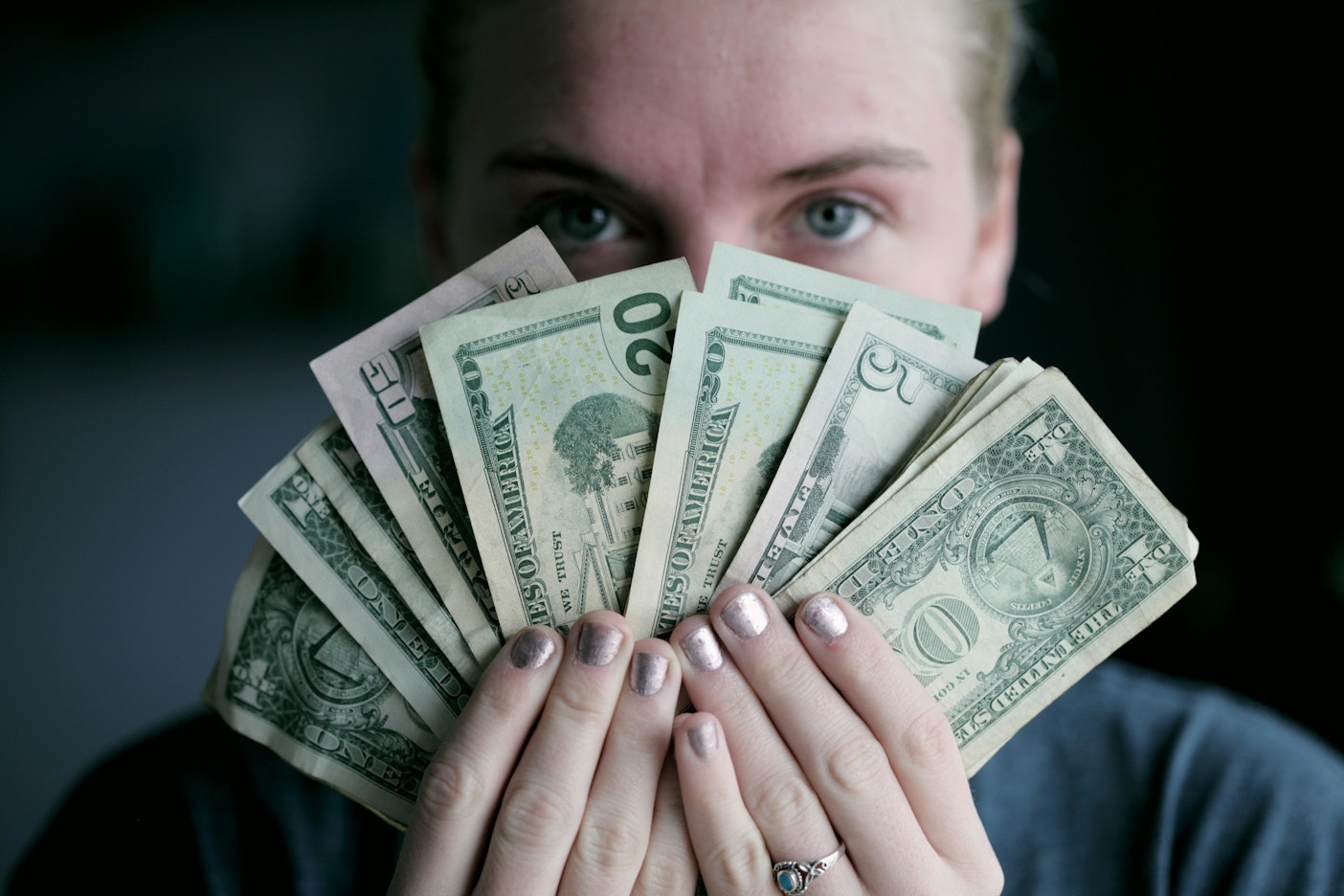
What is Pricing Technology in the Insurance Industry?
Pricing technology in the insurance industry is revolutionizing how insurers set premiums and manage risk. It leverages advanced tools and techniques such as data analytics, machine learning (ML), artificial intelligence (AI), and actuarial models. These technologies allow insurers to forecast risk more accurately, analyze customer behavior, and adjust pricing dynamically in response to market conditions.
Gone are the days of relying solely on traditional methods of pricing based on historical trends. Now, insurers can analyze vast amounts of data to identify patterns and predict future risks. AI-driven technologies enable more precise risk modeling, which is essential for determining the right premiums. These tools also help companies stay competitive by adjusting prices in real-time based on changing market conditions.
Three Layers of AI-driven Pricing Technology
The integration of AI into pricing technology can be broken down into three distinct layers, each contributing to more sophisticated and accurate pricing strategies.
The first layer is data collection and integration. Insurers today gather data from a wide array of sources, including historical claims, customer demographics, behavioral data, and external factors like weather and traffic patterns. Predictive analytics, which analyze these data sets, allow insurers to forecast risks and predict the likelihood of claims, helping them set premiums that reflect these future events.
The second layer is the use of machine learning models. These models continuously refine predictions by learning from new data, identifying patterns, and improving pricing accuracy. The more data the system processes, the better it becomes at predicting future risk and adjusting pricing accordingly. This allows insurers to offer more personalized premiums, which can enhance customer satisfaction and improve retention.
The third layer involves dynamic pricing systems. These systems enable insurers to make real-time adjustments to premiums based on factors such as market trends, individual customer data, and competitive forces. The ability to adjust pricing in real time ensures that insurers can stay competitive in the marketplace while maximizing profitability.
Premium Optimization
Premium optimization takes pricing technology a step further by focusing on finding the "optimal" price point. This is the sweet spot where insurers can balance profitability with customer retention and acquisition. The process begins with risk assessment, where predictive algorithms assess the risk associated with each policyholder. Insurers use these assessments to set premiums that reflect the risk each individual poses.
Customer segmentation plays a key role in premium optimization. Customers are grouped based on factors such as their risk profile, behavior, and willingness to pay. This segmentation allows insurers to offer personalized pricing, which is increasingly becoming a key driver of customer satisfaction. By offering tailored premiums, insurers can ensure they are competitive and avoid alienating customers with generalized pricing models.
Elasticity analysis is another important component of premium optimization. Pricing models estimate how sensitive customers are to price changes, which helps insurers identify the thresholds for maximizing conversions without compromising profitability. Insurers also use simulations and scenarios to test various pricing strategies and evaluate their impact on revenue and customer retention before deploying them.
A continuous feedback loop is established, where pricing models are updated with new data and outcomes. This ensures that insurers are continuously refining their pricing strategies, adapting to changes in customer behavior, and keeping pace with market trends.
Benefits of AI-driven Pricing Technology vs. Traditional Pricing Models

AI-driven pricing technology offers several advantages over traditional pricing models. In traditional pricing, premiums are often set based on centralized decision-making, using regression and linear models. These models, while effective in the past, have limitations when it comes to analyzing complex, non-linear factors that influence risk and customer behavior.
In contrast, AI-driven pricing uses dynamic, real-time adjustments and non-linear models that incorporate a broader range of variables. This allows insurers to not only assess risk more accurately but also to offer personalized pricing that better matches customer expectations. AI-driven models also support more granular price discrimination, ensuring that premiums are adjusted to reflect the individual characteristics of each customer.
The key benefits of AI-driven pricing technology include improved accuracy, which reduces the chances of underpricing or overpricing; competitive advantage, as dynamic pricing helps insurers stay ahead in competitive markets; customer retention, with personalized pricing leading to higher customer satisfaction; and operational efficiency, as automation accelerates pricing processes and reduces manual effort.
Challenges
Despite the clear advantages, there are several challenges that come with the adoption of AI in pricing. One of the biggest challenges is data privacy and regulation. Collecting and analyzing sensitive customer data requires strict adherence to data protection laws. Ensuring that pricing models comply with these regulations is critical.
Another challenge is model interpretability. Insurers must ensure that pricing algorithms are transparent and understandable, especially in regulated industries like insurance. This is important not only for regulatory compliance but also for maintaining customer trust.
Finally, bias and fairness are key concerns. If algorithms are not properly designed, they can inadvertently lead to discriminatory pricing. Insurers must work to ensure that their AI models are free from biases that could unfairly impact certain customer segments.
Conclusion
In conclusion, pricing technology is transforming the insurance industry by enabling insurers to set and optimize premiums with greater precision. By using AI-driven tools and dynamic pricing systems, insurers can balance profitability with customer retention while adapting to market demands. Despite the challenges, the benefits of pricing technology—such as improved accuracy, competitive advantage, and customer satisfaction—make it an essential part of the future of insurance.
Subscribe to Our Newsletter
Get the latest insights about Global solutions for leading insurers on your email